Bayesian Structural Time Series Models for Predicting the \(>_2\) Emissions in Afghanistan
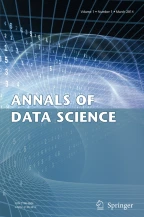
There are numerous forecasting methods, and these approaches take only data, analyse it, produce a prediction by analysing, ignore the prior information side, and do not take into account the variations that occur over time. The Bayesian structural time series (BSTS) models are the best way to forecast \(>_2\) emissions and is updated. Because \(>_2\) emissions play an essential part in climate change, forecasting future \(>_2\) emissions is critical for all countries where global warming is a hazard to the planet. This study models and forecasts \(>_2\) emissions in Afghanistan from 1990 to 2019 using the BSTS models, bsts function from the bsts R package statistical tool. We did a diagnostics test of the normality of the residuals out of the bsts R package . According to the findings for 12 years ahead, \(>_2\) emissions will rise by 2031 in all models findings. The study’s findings indicate that \(>_2\) emissions in Afghanistan are projected to rise, exposing the country to climate-related concerns.
This is a preview of subscription content, log in via an institution to check access.
Access this article
Subscribe and save
Springer+ Basic
€32.70 /Month
- Get 10 units per month
- Download Article/Chapter or eBook
- 1 Unit = 1 Article or 1 Chapter
- Cancel anytime
Buy Now
Price includes VAT (France)
Instant access to the full article PDF.
Rent this article via DeepDyve
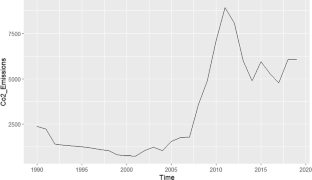
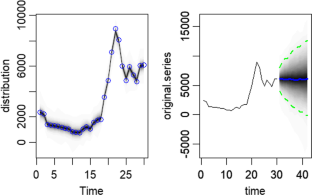
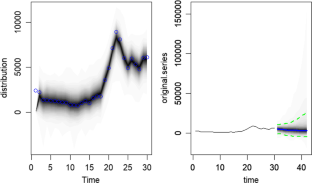
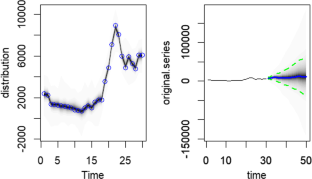
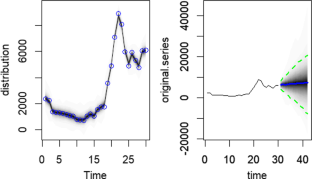
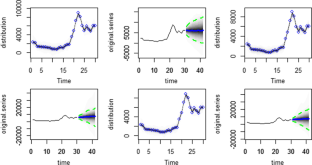
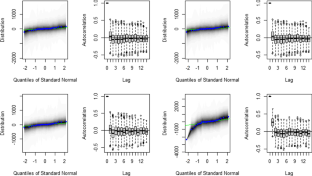
Similar content being viewed by others
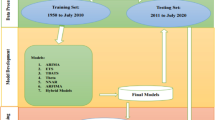
Exploring hybrid models for forecasting \(CO_2\) emissions in drought-prone Somalia: a comparative analysis
Article 31 October 2023
Persistence, Mean-Reversion and Non-linearities in \(\hbox \) Emissions: Evidence from the BRICS and G7 Countries
Article 25 February 2016
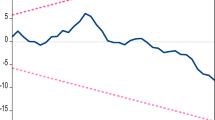
Modeling CO2 emissions in Malaysia: an application of Maki cointegration and wavelet coherence tests
Article 22 January 2021
Explore related subjects
Data Availability
The manuscript has a data link.
References
- Olson DL, Shi Y, Shi Y (2007) Introduction to business data mining, vol 10. McGraw-Hill/Irwin, New York, pp 2250–2254 Google Scholar
- Shi Y (2022) Advances in big data analytics. Adv Big Data Anal
- Tien JM (2017) Internet of things, real-time decision making, and artificial intelligence. Ann Data Sci 4:149–178 ArticleGoogle Scholar
- Shi Y, Tian Y, Kou G, Peng Y, Li J (2011) Optimization based data mining: theory and applications. Springer, Berlin BookGoogle Scholar
- Hossain A, Islam MA, Kamruzzaman M, Khalek MA, Ali MA (2017) Forecasting carbon dioxide emissions in Bangladesh using Box–Jenkins ARIMA models. University of Rajshahi, Department of Statistics, Rajshahi Google Scholar
- Bonga WG, Chirowa F (2014) Level of cooperativeness of individuals to issues of energy conservation. Social Science Research Network. http://ssrn.com/abstract=2412639
- Dietz TA, Ros E (1997) Effects of population and affluence on CO2 emissions. Ecology 175–179
- Putman MW, Ott L, Darmenov A, DaSilva A (2016) A global perspective of atmospheric carbon dioxide concentrations. Parallel Comput 2–8
- Mohammadi A, Burhan A, Mangal R (2020) Impact of population and economic growth on CO2 emission (Case of Afghanistan)
- Abdullah LP, Pauzi HM (2015) Methods in forecasting carbon dioxide emissions: a decade review. J Teknol (Sci Eng) 75(1):67–82 Google Scholar
- Bouznit M, Pablo-Romero MDP (2016) CO2 emission and economic growth in Algeria. Energy Policy 96:93–104 ArticleGoogle Scholar
- Abdullah L, Pauzi HM (2015) Methods in forecasting carbon dioxide emissions: a decade review. J Teknol (Sci Eng) 75(1):67–82 Google Scholar
- Lotfalipour MR, Falahi MA, Bastam M (2013) Prediction of CO2 emissions in Iran using Grey and ARIMA models. Int J Energy Econ Policy 3(3):229–237 Google Scholar
- Basak P, Nandi S (2014) An analytical study of emission dynamics of carbon dioxide in India. IOSR J. Appl. Chem. 16–21
- Hossain A, Islam MA, Kamruzzaman M, Khalek MA, Ali MA (2017) Forecasting carbon dioxide emissions in Bangladesh using Box–Jenkins ARIMA models. University of Rajshahi, Department of Statistics
- Pruethsan S (2017) VARIMAX model to forecast the emission of carbon dioxide from energy consumption in rubber and petroleum industries sectors in Thailand. J Ecol Eng 18(3):112–117 ArticleGoogle Scholar
- Sangeetha A, Amudha T (2018) A novel bio-inspired framework for CO2 emission forecast in India. Procedia Comput Sci 125(2018):367–375 ArticleGoogle Scholar
- Li Y (2020) Forecasting Chinese carbon emissions based on a novel time series prediction method. Energy Sci Eng 8(7):2274–2285 ArticleGoogle Scholar
- Islam MM, Khan MK, Tareque M, Jehan N, Dagar V (2021) Impact of globalization, foreign direct investment, and energy consumption on CO2 emissions in Bangladesh: Does institutional quality matter? Environ Sci Pollut Res 28(35):48851–48871 ArticleGoogle Scholar
- Heydari A, Garcia DA, Keynia F, Bisegna F, De Santoli L (2019) Renewable energies generation and carbon dioxide emission forecasting in microgrids and national grids using GRNN-GWO methodology. Energy Procedia 159:154–159 ArticleGoogle Scholar
- Hosseini SM, Saifoddin A, Shirmohammadi R, Aslani A (2019) Forecasting of CO2 emissions in Iran based on time series and regression analysis. Energy Rep 5:619–631 ArticleGoogle Scholar
- Fatima S, Ali SS, Zia SS, Hussain E, Fraz TR, Khan MS (2019) Forecasting carbon dioxide emission of Asian countries using ARIMA and simple exponential smoothing models. Int J Econ Environ Geol 10(1):64–69 Google Scholar
- Scott SL, Varian HR (2013) Predicting the present with Bayesian structural time series. https://ssrn.com/abstract=2304426 or http://dx.doi.org/10.2139/ssrn.2304426. Accessed on 9 September 2019
- Ross SM (2012) Introduction to probability and statistics for engineers and scientists, 4th edn. Elsevier, Seoul Google Scholar
- Gelman A, Carlin JB, Stern HS, Dunson DB, Vehtari A, Rubin DB (2013) Bayesian data analysis, 3rd edn. Chapman and Hall/CRC Press, Boca Raton BookGoogle Scholar
- Scott SL (2020) Package bsts
- Scott SL (2017) Fitting Bayesian structural time series with the bsts R package. The unofficial Google data science blog
- Almarashi AM, Khan K (2020) Bayesian structural time series. Nanosci Nanotechnol Lett 12(1):54–61
Funding
The study received no financial support.
Author information
Authors and Affiliations
- Department of Statistics and Operations Research, Aligarh Muslim University, Aligarh, India Sayed Rahmi Khuda Haqbin & Athar Ali Khan
- Sayed Rahmi Khuda Haqbin
You can also search for this author in PubMed Google Scholar
You can also search for this author in PubMed Google Scholar
Contributions
Each author contributed equally. The manuscript was written, reviewed, and edited collaboratively by all contributors.
Corresponding author
Ethics declarations
Conflict of interest
Potential conflict of interest All of the writers disclose that they have no competing interests.
Ethical approval
The authors did not copy this work from any source, and it does not harm humans or society.
Additional information
Publisher's Note
Springer Nature remains neutral with regard to jurisdictional claims in published maps and institutional affiliations.
Rights and permissions
Springer Nature or its licensor (e.g. a society or other partner) holds exclusive rights to this article under a publishing agreement with the author(s) or other rightsholder(s); author self-archiving of the accepted manuscript version of this article is solely governed by the terms of such publishing agreement and applicable law.
About this article
Cite this article
Haqbin, S.R.K., Khan, A.A. Bayesian Structural Time Series Models for Predicting the \(>_2\) Emissions in Afghanistan. Ann. Data. Sci. (2024). https://doi.org/10.1007/s40745-023-00510-3
- Received : 05 July 2023
- Revised : 20 November 2023
- Accepted : 07 December 2023
- Published : 24 January 2024
- DOI : https://doi.org/10.1007/s40745-023-00510-3
Share this article
Anyone you share the following link with will be able to read this content:
Get shareable link
Sorry, a shareable link is not currently available for this article.
Copy to clipboard
Provided by the Springer Nature SharedIt content-sharing initiative
Keywords
- Bayesian structural time series (BSTS)
- BSTS R package
- Bayesian inference
- Prior \(>_2\) emissions