Writing the Data Analysis Plan
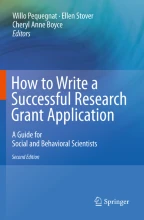
You and your project statistician have one major goal for your data analysis plan: You need to convince all the reviewers reading your proposal that you would know what to do with your data once your project is funded and your data are in hand. The data analytic plan is a signal to the reviewers about your ability to score, describe, and thoughtfully synthesize a large number of variables into appropriately-selected quantitative models once the data are collected. Reviewers respond very well to plans with a clear elucidation of the data analysis steps – in an appropriate order, with an appropriate level of detail and reference to relevant literatures, and with statistical models and methods for that map well into your proposed aims. A successful data analysis plan produces reviews that either include no comments about the data analysis plan or better yet, compliments it for being comprehensive and logical given your aims. This chapter offers practical advice about developing and writing a compelling, “bullet-proof” data analytic plan for your grant application.
This is a preview of subscription content, log in via an institution to check access.
Access this chapter
Subscribe and save
Springer+ Basic
€32.70 /Month
- Get 10 units per month
- Download Article/Chapter or eBook
- 1 Unit = 1 Article or 1 Chapter
- Cancel anytime
Buy Now
Price includes VAT (France)
eBook EUR 93.08 Price includes VAT (France)
Softcover Book EUR 116.04 Price includes VAT (France)
Tax calculation will be finalised at checkout
Purchases are for personal use only
Similar content being viewed by others
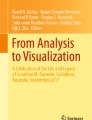
A Holistic Approach to Empirical Analysis: The Insignificance of P, Hypothesis Testing and Statistical Significance*
Chapter © 2020
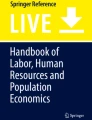
Meta-Analysis
Chapter © 2022
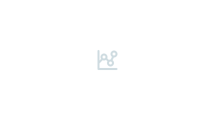
Researchers’ data analysis choices: an excess of false positives?
Article 04 September 2021
References
- Aiken, L. S. & West, S. G. (1991). Multiple regression: testing and interpreting interactions. Newbury Park, CA: Sage. Google Scholar
- Aiken, L. S., West, S. G., & Millsap, R. E. (2008). Doctoral training in statistics, measurement, and methodology in psychology: Replication and extension of Aiken, West, Sechrest and Reno’s (1990) survey of PhD programs in North America. American Psychologist, 63, 32–50. ArticlePubMedGoogle Scholar
- Allison, P. D. (2003). Missing data techniques for structural equation modeling. Journal of Abnormal Psychology, 112, 545–557. ArticlePubMedGoogle Scholar
- American Psychological Association (APA) Task Force to Increase the Quantitative Pipeline (2009). Report of the task force to increase the quantitative pipeline. Washington, DC: American Psychological Association. Google Scholar
- Bauer, D. & Curran, P. J. (2004). The integration of continuous and discrete latent variables: Potential problems and promising opportunities. Psychological Methods, 9, 3–29. ArticlePubMedGoogle Scholar
- Bollen, K. A. (1989). Structural equations with latent variables. New York: Wiley. Google Scholar
- Bollen, K. A. & Curran, P. J. (2007). Latent curve models: A structural equation modeling approach. New York: Wiley. Google Scholar
- Cohen, J., Cohen, P., West, S. G., & Aiken, L. S. (2003). Multiple correlation/regression for the behavioral sciences (3rd ed.). Mahwah, NJ: Erlbaum. Google Scholar
- Curran, P. J., Bauer, D. J., & Willoughby, M. T. (2004). Testing main effects and interactions in hierarchical linear growth models. Psychological Methods, 9, 220–237. ArticlePubMedGoogle Scholar
- Embretson, S. E. & Reise, S. P. (2000). Item response theory for psychologists. Mahwah, NJ: Erlbaum. Google Scholar
- Enders, C. K. (2006). Analyzing structural equation models with missing data. In G. R. Hancock & R. O. Mueller (Eds.), Structural equation modeling: A second course (pp. 313–342). Greenwich, CT: Information Age. Google Scholar
- Hosmer, D. & Lemeshow, S. (1989). Applied logistic regression. New York: Wiley. Google Scholar
- Hoyle, R. H. & Panter, A. T. (1995). Writing about structural equation models. In R. H. Hoyle (Ed.), Structural equation modeling: Concepts, issues, and applications (pp. 158–176). Thousand Oaks: Sage. Google Scholar
- Kaplan, D. & Elliott, P. R. (1997). A didactic example of multilevel structural equation modeling applicable to the study of organizations. Structural Equation Modeling, 4, 1–23. ArticleGoogle Scholar
- Lanza, S. T., Collins, L. M., Schafer, J. L., & Flaherty, B. P. (2005). Using data augmentation to obtain standard errors and conduct hypothesis tests in latent class and latent transition analysis. Psychological Methods, 10, 84–100. ArticlePubMedGoogle Scholar
- MacKinnon, D. P. (2008). Introduction to statistical mediation analysis. Mahwah, NJ: Erlbaum. Google Scholar
- Maxwell, S. E. (2004). The persistence of underpowered studies in psychological research: Causes, consequences, and remedies. Psychological Methods, 9, 147–163. ArticlePubMedGoogle Scholar
- McCullagh, P. & Nelder, J. (1989). Generalized linear models. London: Chapman and Hall. Google Scholar
- McDonald, R. P. & Ho, M. R. (2002). Principles and practices in reporting structural equation modeling analyses. Psychological Methods, 7, 64–82. ArticlePubMedGoogle Scholar
- Messick, S. (1989). Validity. In R. L. Linn (Ed.), Educational measurement (3rd ed., pp. 13–103). New York: Macmillan. Google Scholar
- Muthén, B. O. (1994). Multilevel covariance structure analysis. Sociological Methods & Research, 22, 376–398. ArticleGoogle Scholar
- Muthén, B. (2008). Latent variable hybrids: overview of old and new models. In G. R. Hancock & K. M. Samuelsen (Eds.), Advances in latent variable mixture models (pp. 1–24). Charlotte, NC: Information Age. Google Scholar
- Muthén, B. & Masyn, K. (2004). Discrete-time survival mixture analysis. Journal of Educational and Behavioral Statistics, 30, 27–58. ArticleGoogle Scholar
- Muthén, L. K. & Muthén, B. O. (2004). Mplus, statistical analysis with latent variables: User’s guide. Los Angeles, CA: Muthén &Muthén. Google Scholar
- Peugh, J. L. & Enders, C. K. (2004). Missing data in educational research: a review of reporting practices and suggestions for improvement. Review of Educational Research, 74, 525–556. ArticleGoogle Scholar
- Preacher, K. J., Curran, P. J., & Bauer, D. J. (2006). Computational tools for probing interaction effects in multiple linear regression, multilevel modeling, and latent curve analysis. Journal of Educational and Behavioral Statistics, 31, 437–448. ArticleGoogle Scholar
- Preacher, K. J., Curran, P. J., & Bauer, D. J. (2003, September). Probing interactions in multiple linear regression, latent curve analysis, and hierarchical linear modeling: Interactive calculation tools for establishing simple intercepts, simple slopes, and regions of significance [Computer software]. Available from http://www.quantpsy.org.
- Preacher, K. J., Rucker, D. D., & Hayes, A. F. (2007). Addressing moderated mediation hypotheses: Theory, methods, and prescriptions. Multivariate Behavioral Research, 42, 185–227. ArticleGoogle Scholar
- Raudenbush, S. W. & Bryk, A. S. (2002). Hierarchical linear models: Applications and data analysis methods (2nd ed.). Thousand Oaks, CA: Sage. Google Scholar
- Radloff, L. (1977). The CES-D scale: A self-report depression scale for research in the general population. Applied Psychological Measurement, 1, 385–401. ArticleGoogle Scholar
- Rosenberg, M. (1965). Society and the adolescent self-image. Princeton, NJ: Princeton University Press. Google Scholar
- Schafer. J. L. & Graham, J. W. (2002). Missing data: Our view of the state of the art. Psychological Methods, 7, 147–177. ArticlePubMedGoogle Scholar
- Schumacker, R. E. (2002). Latent variable interaction modeling. Structural Equation Modeling, 9, 40–54. ArticleGoogle Scholar
- Schumacker, R. E. & Lomax, R. G. (2004). A beginner’s guide to structural equation modeling. Mahwah, NJ: Erlbaum. Google Scholar
- Selig, J. P. & Preacher, K. J. (2008, June). Monte Carlo method for assessing mediation: An interactive tool for creating confidence intervals for indirect effects [Computer software]. Available from http://www.quantpsy.org.
- Singer, J. D. & Willett, J. B. (1991). Modeling the days of our lives: Using survival analysis when designing and analyzing longitudinal studies of duration and the timing of events. Psychological Bulletin, 110, 268–290. ArticleGoogle Scholar
- Singer, J. D. & Willett, J. B. (1993). It’s about time: Using discrete-time survival analysis to study duration and the timing of events. Journal of Educational Statistics, 18, 155–195. ArticleGoogle Scholar
- Singer, J. D. & Willett, J. B. (2003). Applied longitudinal data analysis: Modeling change and event occurrence. New York: Oxford University. BookGoogle Scholar
- Vandenberg, R. J. & Lance, C. E. (2000). A review and synthesis of the measurement invariance literature: Suggestions, practices, and recommendations for organizational research. Organizational Research Methods, 3, 4–69. ArticleGoogle Scholar
- Wirth, R. J. & Edwards, M. C. (2007). Item factor analysis: Current approaches and future directions. Psychological Methods, 12, 58–79. ArticlePubMedCASGoogle Scholar
Author information
Authors and Affiliations
- L. L. Thurstone Psychometric Laboratory, Department of Psychology, University of North Carolina, Chapel Hill, NC, USA A. T. Panter
- A. T. Panter
You can also search for this author in PubMed Google Scholar
Corresponding author
Editor information
Editors and Affiliations
- National Institute of Mental Health, Executive Blvd. 6001, Bethesda, 20892-9641, Maryland, USA Willo Pequegnat
- National Institute of Mental Health, Executive Blvd. 6001, Bethesda, 20892-9641, Maryland, USA Ellen Stover
- Delafield Place, N.W. 1413, Washington, 20011, District of Columbia, USA Cheryl Anne Boyce
Rights and permissions
Copyright information
© 2010 Springer Science+Business Media, LLC
About this chapter
Cite this chapter
Panter, A.T. (2010). Writing the Data Analysis Plan. In: Pequegnat, W., Stover, E., Boyce, C. (eds) How to Write a Successful Research Grant Application. Springer, Boston, MA. https://doi.org/10.1007/978-1-4419-1454-5_22
Download citation
- DOI : https://doi.org/10.1007/978-1-4419-1454-5_22
- Published : 20 August 2010
- Publisher Name : Springer, Boston, MA
- Print ISBN : 978-1-4419-1453-8
- Online ISBN : 978-1-4419-1454-5
- eBook Packages : MedicineMedicine (R0)
Share this chapter
Anyone you share the following link with will be able to read this content:
Get shareable link
Sorry, a shareable link is not currently available for this article.
Copy to clipboard
Provided by the Springer Nature SharedIt content-sharing initiative